2017年12月20日下午14点,应广州大学经济与统计学院和岭南统计科学研究中心的邀请,英国伦敦经济与政治科学学院姚琦伟教授在行政东前座412会议室作了题为“Principal Component Analysis for Vector Time Series”的讲座——暨“羊城讲坛”第二十讲,旨在进一步提高年轻学者及研究生对研究的理解。此次讲座由系主任张兴发主持,相关专业的师生参加了此次讲座。本报告讲述将主成分分析(PCA)扩展到二阶平稳矢量时间序列,这是因为我们寻求一个p-变量时间序列的临时线性变换,使得变换后的序列被分割成几个较低维的子序列,而那些子序列彼此同时并且连续地不相关。因此,就线性动态结构而言,可以分别分析这些较低维数的系列。
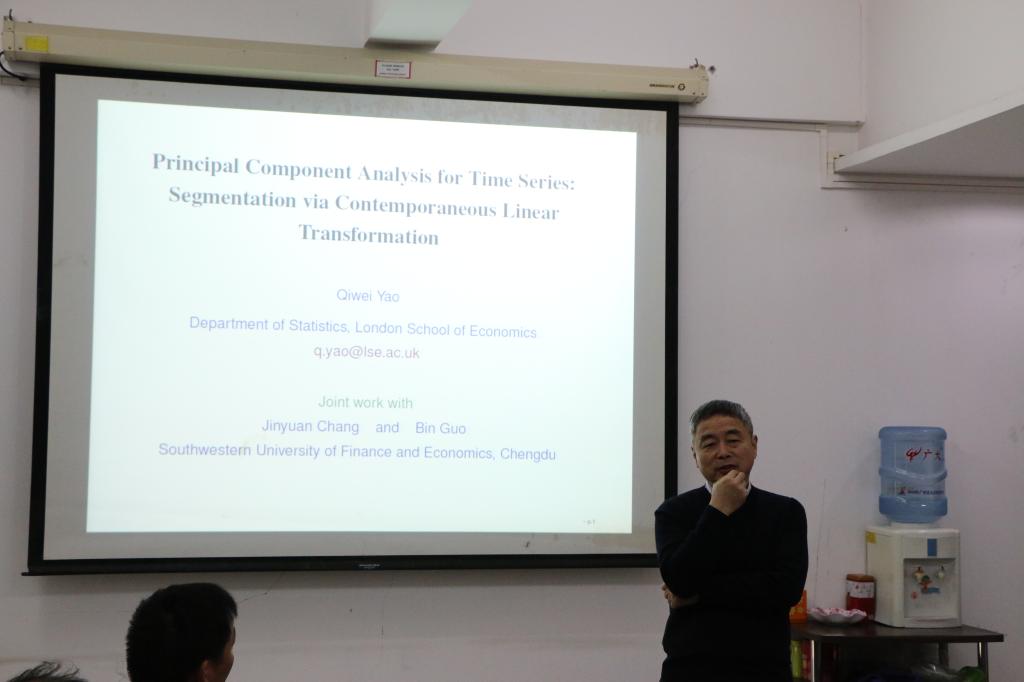
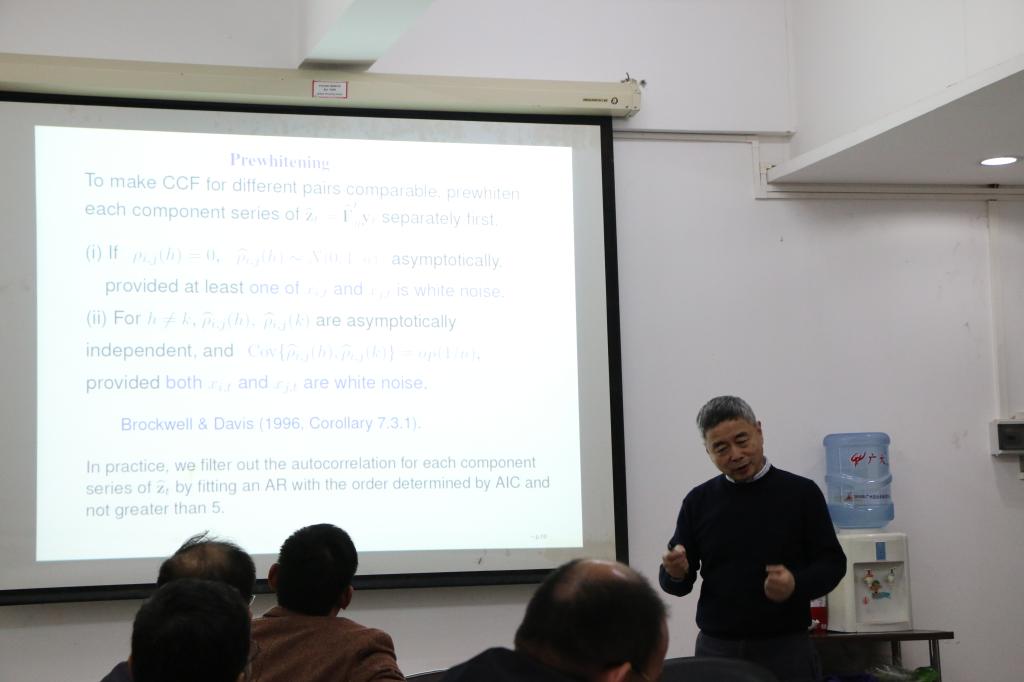
摘要:We extend the principal component analysis (PCA) to second-order stationary vector time series in the sense that we seek for acontemporaneous linear transformation for a p-variate time series such that the transformed series is segmented into several lower-dimensionalsubseries, and those subseries are uncorrelated with each other both contemporaneously and serially. Therefore those lower-dimensionalseries can be analysed separately as far as the linear dynamic structureis concerned. Technically it boils down to an eigenanalysis for apositive definite matrix. When $p$ is large, an additional step is required to perform a permutation in terms of either maximum cross-correlations or FDR based on multiple tests. The asymptotictheory is established for both fixed p diverging p when the sample size n tends to infinity. Numerical experiments with both simulated and real datasets indicate that the proposed method is aneffective initial step in analysing multiple time series data, which leads to substantial dimension reduction in modelling andforecasting high-dimensional linear dynamical structures. Unlike PCA for independent data, there is no guarantee that the required linear transformation exists. When it does not, the proposed methodprovides an approximate segmentation which leads to the advantages in, for example, forecasting for future values. The method can alsobe adapted to segment multiple volatility processes.
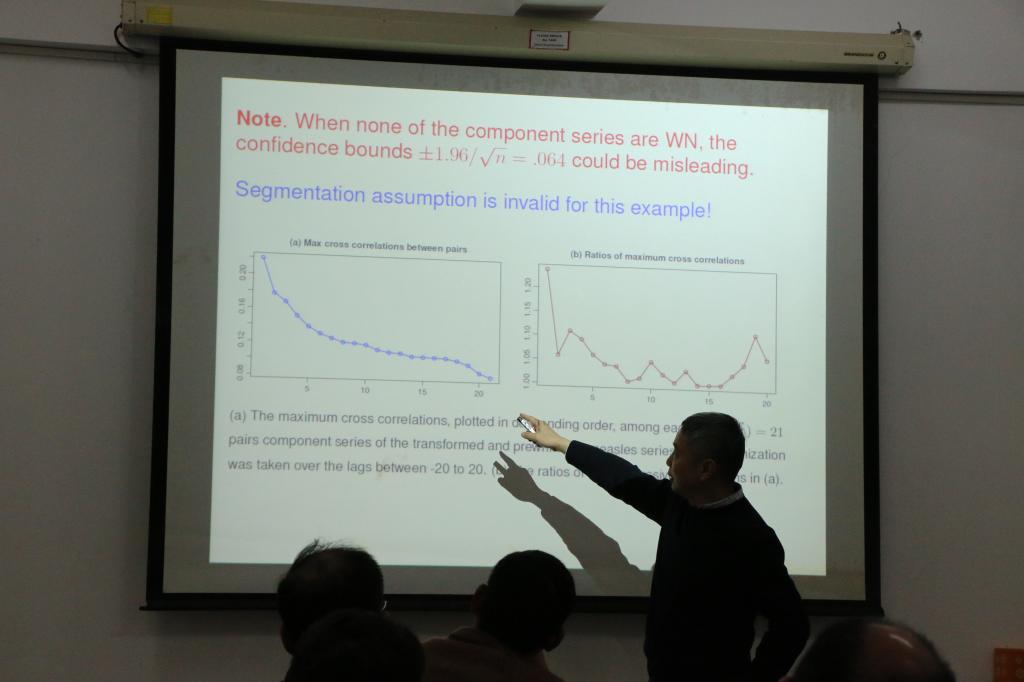